Use Cases
Turn product interactions into powerful insights with Split
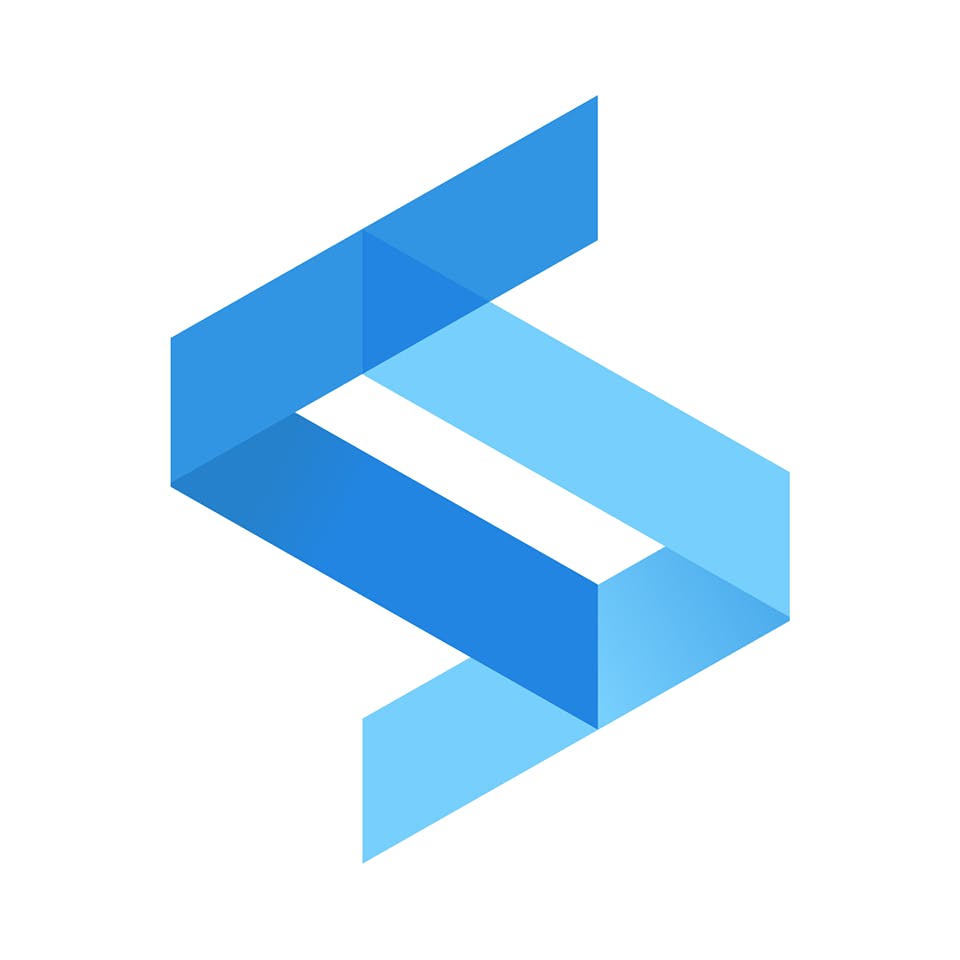
If you want to engage and delight your customers, you need to understand how they use your product's features. By collecting robust on-platform interaction data, you can understand who visits your apps and websites, identify features that help move users through journeys, and diagnose those that present roadblocks.
For product teams, this is mission-critical information that helps them eliminate usability problems, minimize churn, and deliver personalized user interactions. But what if your feature engagement data could add value beyond the product life cycle? What if you could leverage this information to gain deeper insights about who your customers are, what they want, and how their needs may change in the future?
This use case walks you through how to use Split, mParticle, and your data warehousing tool of choice to incorporate your on-platform data into organization’s broad strategic initiatives.
STEP 1: Implement feature flags in Split
Split feature flags are if/else statements in a product’s code that (among many other capabilities) allow product stakeholders to determine the features a user will see in real time. As a first step, implement Split feature flags across your product to support your product analytics goals.
STEP 2: Send feature interaction data from Split to mParticle
In addition to helping you direct user journeys in real time, you can also leverage Split feature flags to build a record of how your customers interact with your products through “impressions”––event objects that contain metadata about feature interactions. Once you have set up your integration between Split and mParticle, you can set impressions as an event output and send this data from the Split platform as a feed input in mParticle.
STEP 3: Send your Split interaction data to your favorite Data Warehousing and BI tools
With Split impressions flowing into mParticle, you can easily send this information as a raw data export to your favorite data warehouses, such as Amazon Redshift, Google BigQuery, and Snowflake, where it can then be queried by a BI tool, such as Looker. Combining these feature engagement records with the user events captured directly through mParticle, and forwarding all of that data to your data warehouse increases the diversity (and therefore predictive potential) of the raw data that powers your BI insights.