Part Five: The Hidden Cost of Warehouse-Native
This post is part of the CDP 2.0: Why Zero-Waste Is Now series. For the best experience, we recommend starting with the Introduction and reading each chapter in sequence.
At mParticle, we have been highly focused on supporting our customers’ data quality and governance efforts for the past number of years, and have extended this to support "zero-copy" strategies. We will be deploying our own CDW overlay architecture specifically for this purpose.
While we acknowledge that data storage optimization is important for governance, we believe focusing on optimizing CDP compute costs in order to maximize value for our customers is both a more pressing market need and a much bigger opportunity. We feel this is critical, especially as we consider all of the compute-intensive ML/AI-powered decisioning coming to market in the coming quarters.
Over the last decade, we invested significant resources in continuously optimizing our compute architecture, specifically focused on providing real-time personalization capabilities at scale. It is our belief scaled marketers will require this to activate real-time CDP AI capabilities. Consequently, we have been doing research to determine the optimal compute architectures for these specific use cases. While the warehouse-native approach certainly offers some desirable traits, mainly inheriting the underlying CDW's quality and governance characteristics, they are not highly optimized, nor can be, for scaled real-time applications. Even after our efforts to highly optimize, our research shows the following cost profile for deploying audiences with various refresh latencies:
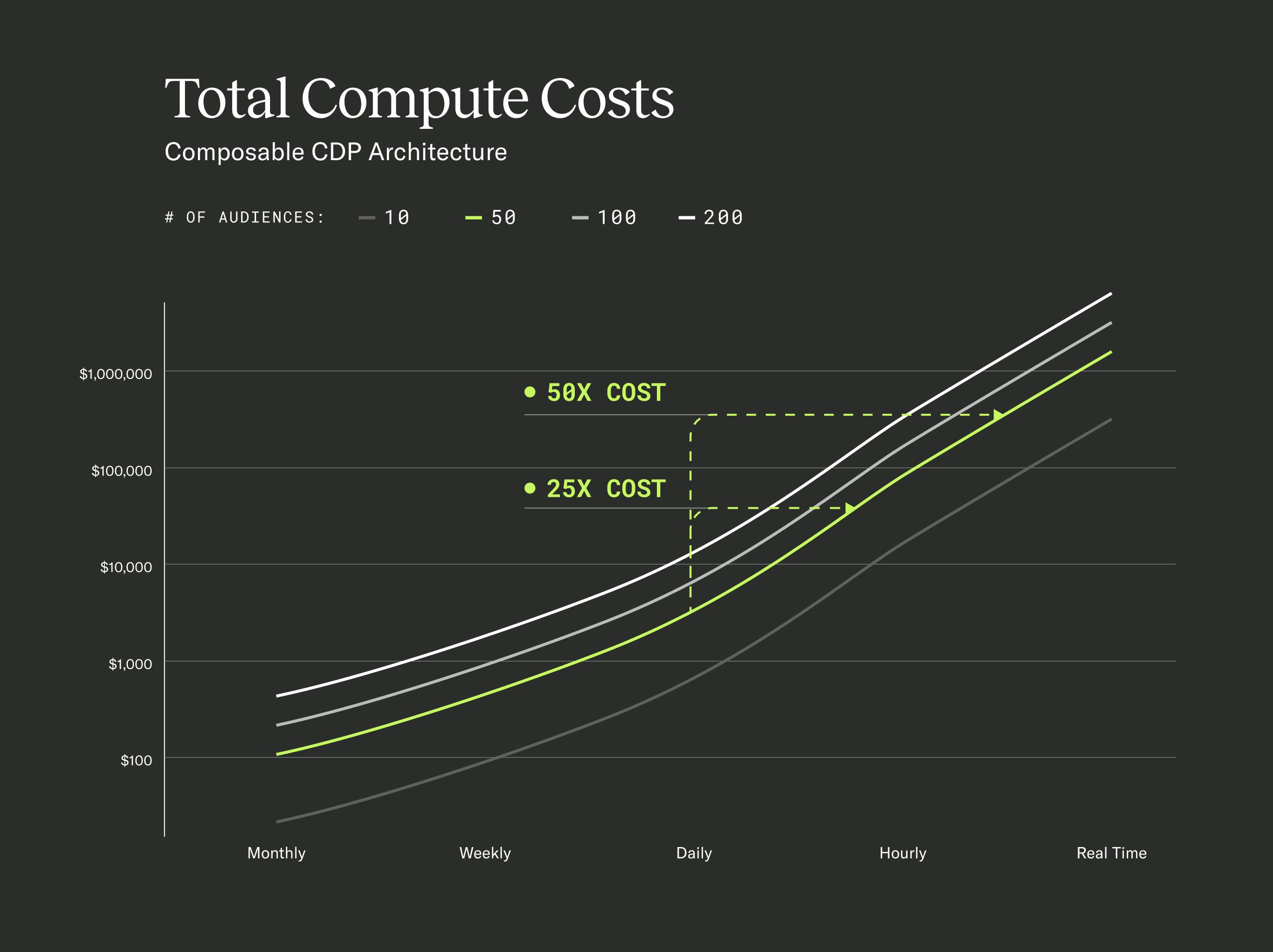
This chart shows moving from audience daily refreshes to hourly refreshes can result in 25x compute cost increases. Moving from daily to five-minute syncs, which are the closest thing offered to real-time refreshes (which is not actually achievable in current warehouse-native architectures), takes costs up at least 50x. Another important observation is the cost acceleration which occurs when improving refresh speeds under 24 hours.
While warehouse-native CDPs benefit from a pricing model which does not have to reflect these costs, their customers do pay them. Many are starting to notice these effects on their CDW compute costs. This will only be exacerbated by ML/AI applications, which are compute heavy. Given our long term vision of an agentic AI CDP, which leverages real-time data signals, we are not convinced current composable architectures are the best approach.
For CDP customers, the main question to consider is whether low latency marketing applications are needed to achieve your strategic goals. Certainly, if you have low consideration transactions, or customer journeys which are highly interactive, this will be the case. More specifically, will you most likely need variety of audience segments which are able to update at the same speed that your customers are engaging? If so, this compute cost profile should be of great interest and investigation, as it would otherwise be a limiting factor on marketing capabilities via a warehouse-native solution.
In the longer term, lakehouse or data mesh architectures will be the main mechanism for addressing compute costs. In these architectures, optimized compute engines access a universal data store, probably based on Delta Lake or Iceberg. An optimized CDP compute engine will be the preferred architecture for advanced marketing applications. This is the roadmap mParticle is pursuing.
While the debate has raged on over the past few years over the merits, the costs, benefits, and tradeoffs of various CDP approaches, we want to provide hard evidence of the cost differential in compute-optimized solutions versus the alternatives. We will be publishing the complete findings in the coming weeks, stay tuned.