Data Master 2.0: Ensure data quality
New Data Master features, now in Early Access, provide teams with an easy way to stop bad data at the source with data frameworks, data validation, and data quality enforcement.
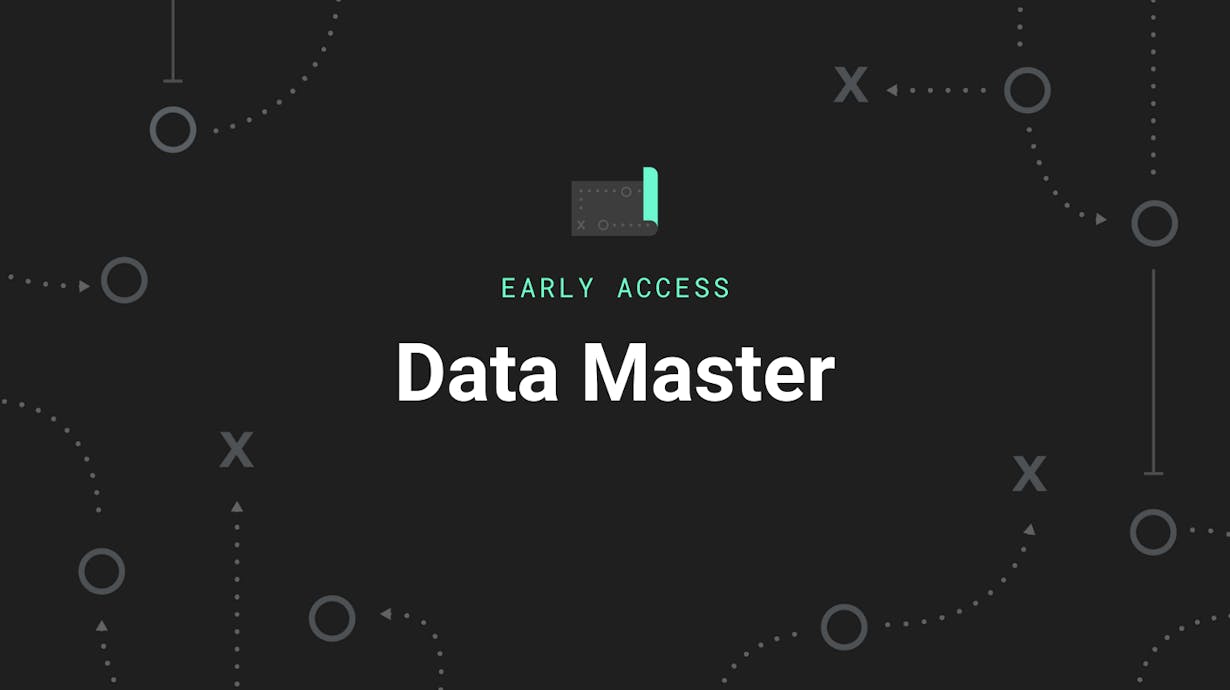
Basing business decisions on reliable, valid data can help you create better customer experiences, improve conversion rates and ROI, and provide your teams with much-needed guidance as they build roadmaps. Unfortunately, as many teams know, ensuring that your data is consistently correct, clean, and valid can be a major challenge, especially as organizations grow and their data scales. To help make bad data a thing of the past, we launched Data Master, which is designed to help align stakeholders around a shared data dictionary and introduce total quality management (TQM) into data processes. But, beyond access to a central view of your data for at-a-glance validation, you also need to be able to ensure that your data strategy stays on track while evolving with your needs as your teams, organization, data sources, and throughput changes over time.
Today, we’re excited to announce the upcoming expansion of Data Master’s platform features, which will provide product managers, marketers, devs, and data scientists with a framework to define, standardize, and govern the way customer data is collected and automatically validated within mParticle to ensure data quality at all times.
The new updates center around three key areas that will help teams shorten their customer data onboarding processes and minimize errors:
- Data Planning
- Data Validation
- Data Quality Enforcement
Data Planning
Bad data increases costs, wastes time, incapacitates decision-making, frustrates customers, and makes it difficult to execute any sort of customer data strategy with confidence.
Data Master will provide our team with a simple way to see all of the data mParticle is collecting in one place, so we can debug and democratize data, and maintain data continuity more easily.
Mobile Product Manager, Chewy.com
With mParticle, teams can now create collaborative Data Plans to collect, manage, and validate data based on a shared agreement and understanding of how it is used, shared, and protected. Along with data validation, data planning ensures that everyone is on the same page when it comes to your data. Data Plans can also help teams understand how customer data evolves over time by identifying and resolving data issues quickly—so you always know you can trust your data and your data sources.
Here’s how a Data Plan works:
- Create a new Data Plan with data points to be collected and their schema definitions
- Download Data Plan in a JSON format to support a build spec so that data stakeholders can ensure that developers follow the expected data standards specifications
- Review how incoming data is faring against your plan and make necessary adjustments
- Update Data Plans as teams collaborate and see a historical changelog for transparency
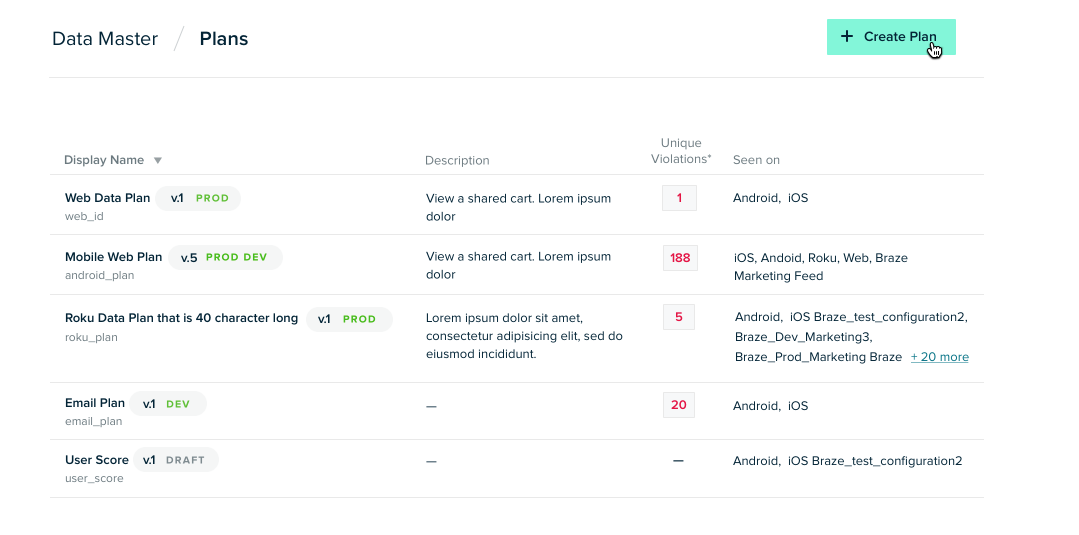
Data Validation
Making sure all team members know what’s going on is essential to a smoothly functioning business process.
Teams can now visually explore everything about their customer data—including data source type, business terms, and errors—in one place, with detailed data validation and schema violations statistics.
Customer data can be validated in two ways:
- Data Catalog allows you to see all of the data mParticle is collecting in one place to debug and maintain data continuity
- Live Stream allows you to identify and resolve data validation issues quickly
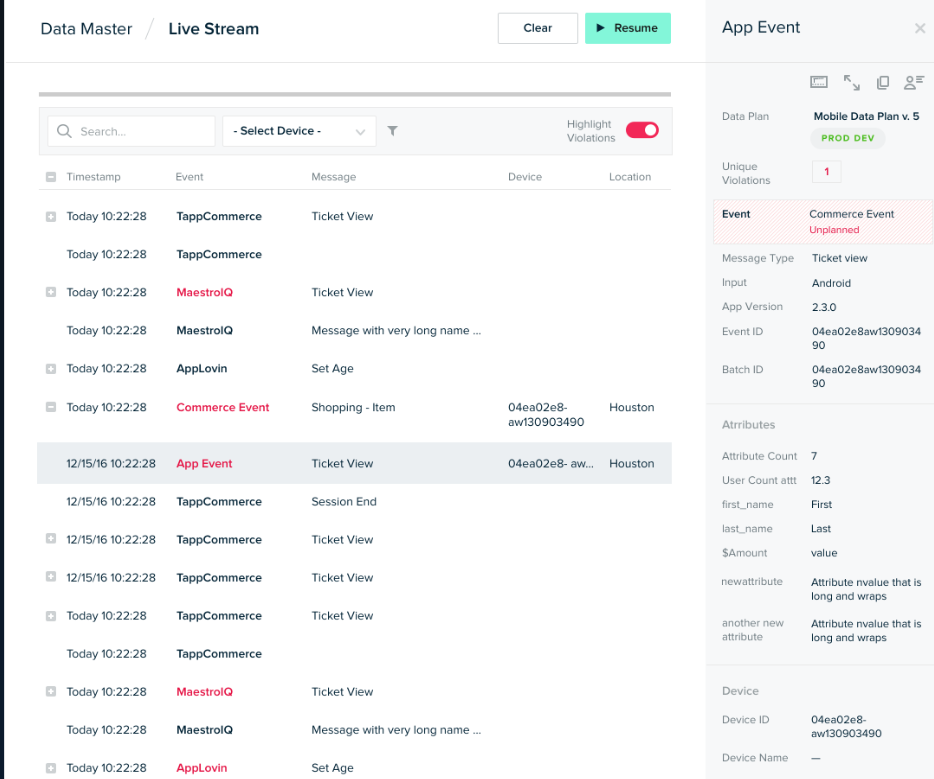
Data Quality Enforcement
Data Quality is not a one-time task, but a continuous process that requires all data stakeholders to be data-focused.
To help you avoid polluting production data, the new data quality enforcement rules allow teams to identify and address erroneous data that does not conform to expectations defined in the Data Plan. The types of errors that can now be mitigated with Data Plans and Data Validation are:
Unexpected data -
Mistakes happen during implementation, whether it's accidentally misspelled data or misunderstood requirements by developers
Incomplete data -
Planned data that was missed during implementation
Human errors -
Human errors, such as attributes coded as a string instead of an array.
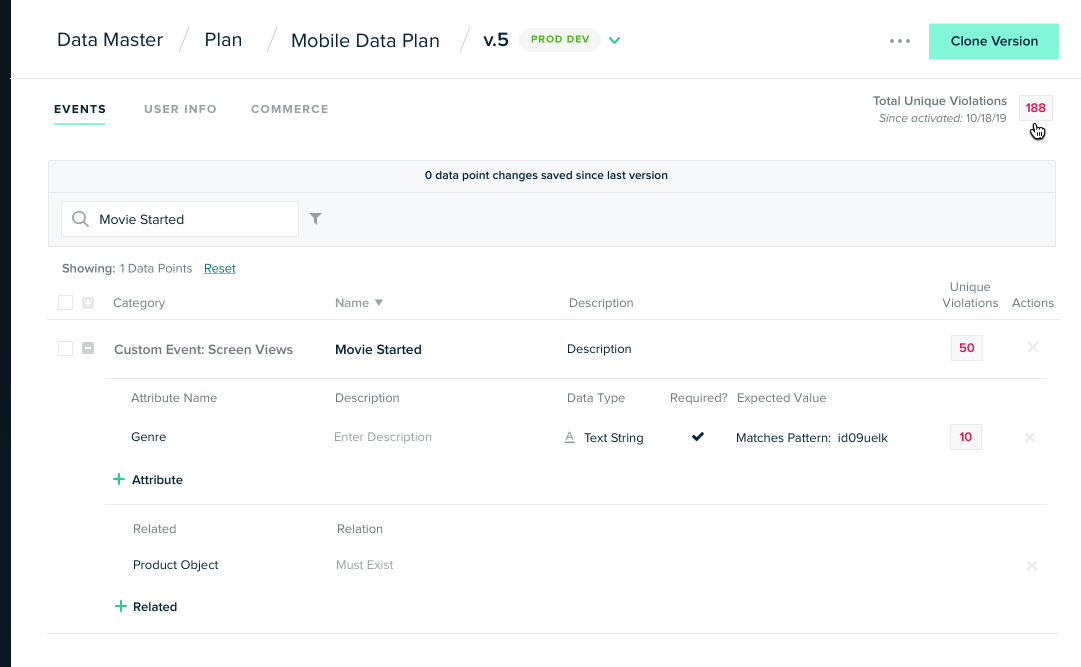
Join the Early Access Program
Interested in participating? Existing customers can contact their mParticle CSM to learn more about this early access program. If you’d like to learn more about Data Master’s existing and new features and what it can do for your data quality, get in touch with us here.