Data enrichment and machine learning: Maximizing the value of your data insights
Data enrichment and machine learning are two techniques that can enhance the ability of your customer data to drive personalized experiences. While there is some overlap in the end goal of both approaches to enhancing data value, there are significant differences in the time, resources, and overhead they each require.
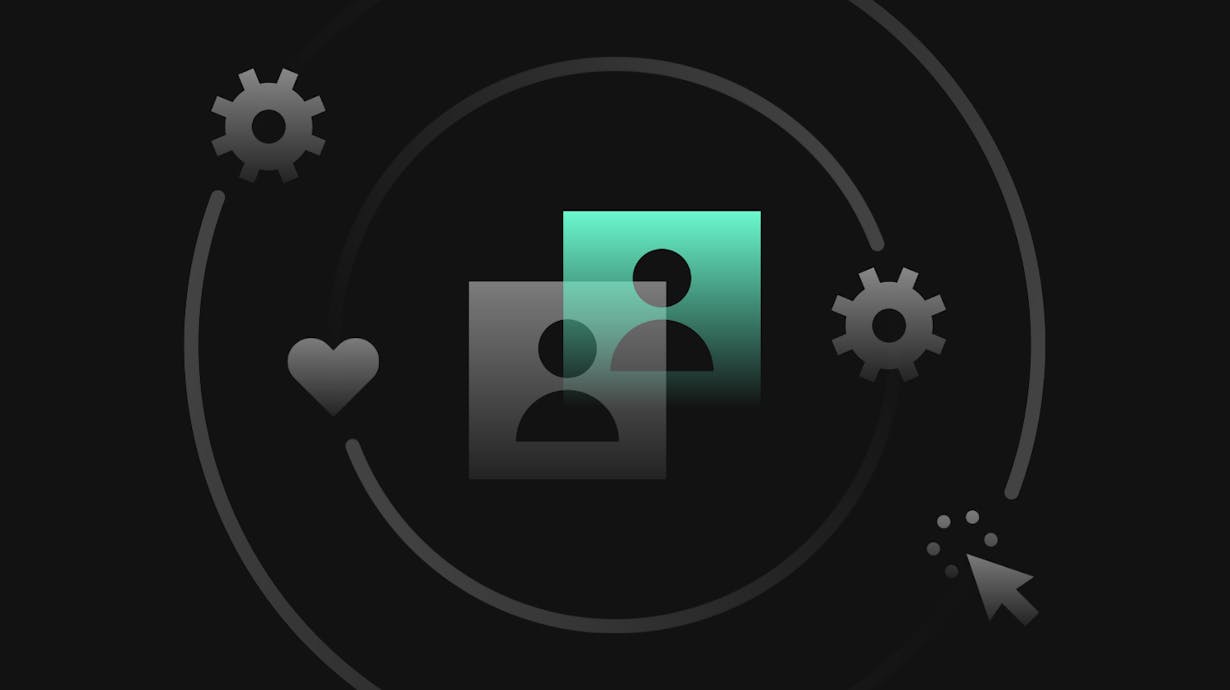
In the last decade, companies of all kinds have seen a dramatic increase in the amount of data they collect, store, and process. In large part, this is thanks to a rapidly expanding ecosystem of software tools that enable companies to analyze and act on this data in new and impactful ways.
Among the different types of data points that companies can collect and leverage, first-party data––which refers to data harvested directly from customers on a company’s websites, apps and other owned properties––is the best and most reliable form of customer data in an organization's arsenal for driving deep, real-time personalization across touchpoints.
Certain use cases like market forecasting, predictive analytics, and inferential recommendations rely on a deeper story than the one first-party data can tell explicitly, however. To meet the needs of these operations, data stakeholders need to reach for tools that allow them to either expand upon the datasets they have collected from owned properties, or look inside their data in a more intelligent way.
Enriching your customer data with third-party datasets and generating machine learning models are two approaches to enhancing your understanding of your customers in a way that serves these complex needs. In many cases, either path can work, though there are important differences in the tools, expertise, and infrastructure required to leverage each approach.
What is data enrichment?
Data enrichment is the process of acquiring second- or third-party data, and using it to augment or cleanse your first-party data. Before we progress, let’s quickly recap the three different categories of customer data:
- First-party data is collected directly from your customers on your own properties.
- Second-party data is another company’s first-party data that you purchase and add to your first-party data.
- Third-party data comes from large data aggregators that pay companies, social networks, and other data owners for their first-party data.
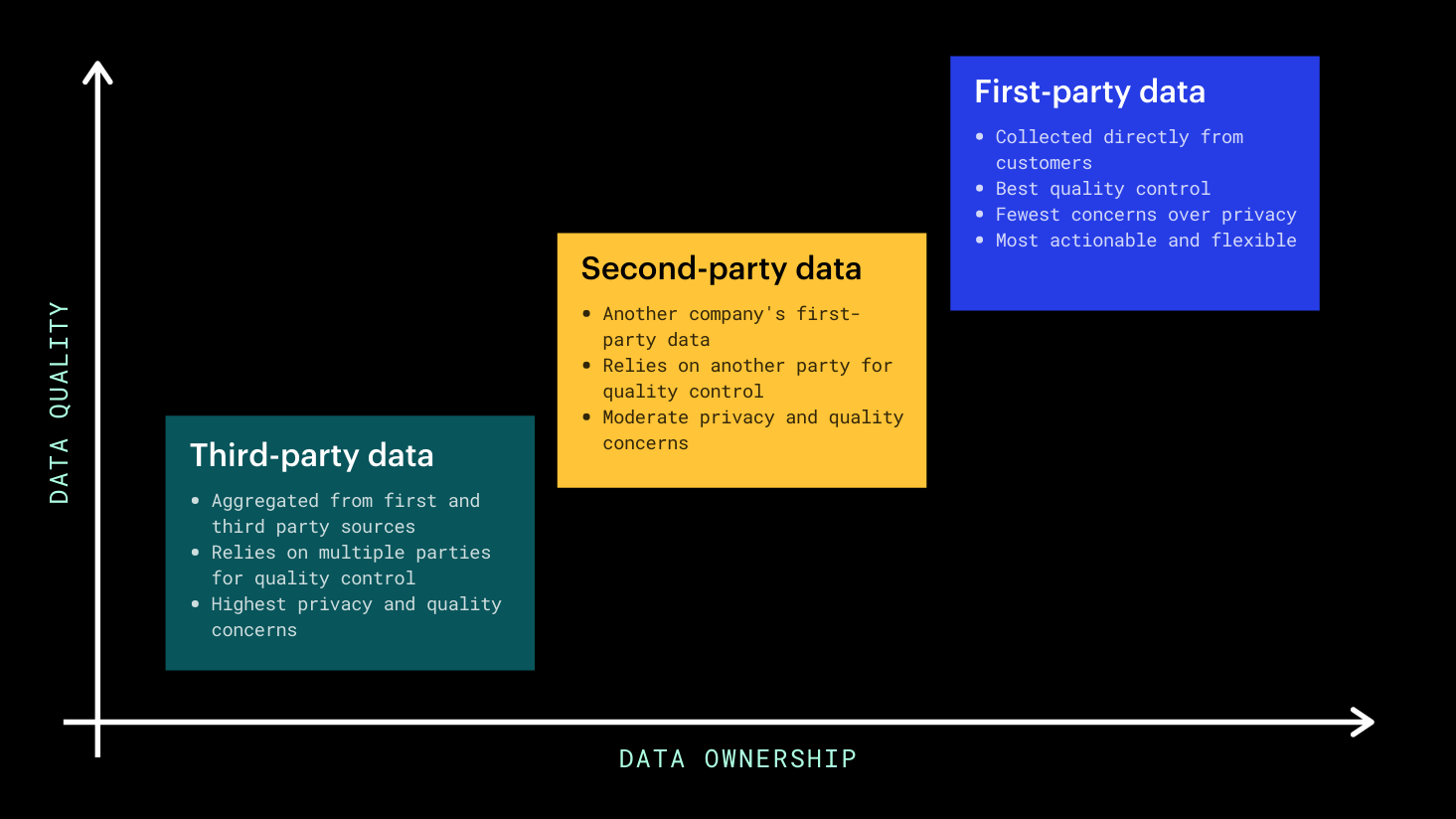
Key differences between categories of customer data.
It should be noted, however, that with an infrastructure Customer Data Platform at the heart of your data stack, data quality poses less of a problem, as consistent schema are maintained within your data from the point of collection. But even if your company benefits from tightly integrated, permanent customer data infrastructure––the gold standard for driving maximum value from your customer data––there are still use cases for which enriching your view of the customer with third-party insights could be beneficial. Here are a few examples:
Customer segmentation
Additional layers of third-party data can help you categorize your customers into more granular interest groups than first-party data alone, and more targeted messaging can uncover new marketing opportunities and growth potential. Say, for example, you want to push emails promoting conference registration to members of a particular professional association. You have captured your prospects’ job titles, company names, and contact information from your landing pages and lead generation forms, but membership in this organization is not a datapoint you currently have. Rather than adding an additional field to your existing forms asking whether your prospects belong to this organization, you can acquire a third-party data set from LinkedIn, add it to your existing customer profiles, and segment your prospects into members and non-members.
Enhanced personalization
Ten years ago, cross-channel personalization may have been “icing on the cake” when it comes to communicating with customers. But now, it is a baseline expectation. In a survey of 6,700 consumers conducted by Salesforce Research revealed what factors determine whether a company will win their business:
- 84% said being treated like a person, not a number, is very important.
- 70% said understanding how they use products and services is very important.
- 56% actively seek to buy from the most innovative companies (i.e. those that consistently introduce new products and services based on customer needs).
The ways in which data enrichment can enhance your ability to personalize communications and messaging across customer touchpoints are many and varied. For example, new B2C companies in early growth stages have not had time to collect robust first-party data sets revealing their potential customers’ purchase habits and tastes when it comes to retail products. By integrating enrichment data from a social network into their existing first-party dataset indicating which similar products and brands their prospects have interacted with, this nascent company can have a much more complete idea of which products and offers to present to their prospects in emails and onsite messaging.
Getting the most out of data enrichment
As more companies are looking to leverage their customer data to the fullest extent, the demand for enrichment datasets is growing rapidly. In turn, the number of third-party data purveyors is similarly expanding at a steady pace. Not all data providers adhere to the same quality assurance standards, however, and it can sometimes be difficult to assess the accuracy of third-party datasets. To help, mParticle created the Data Partners Program––a group of enrichment data partners that meet the highest standard of data quality, all of which integrate seamlessly with mParticle’s CDP. This initiative helps companies select a reliable data enrichment partner, and also makes it easier to integrate enrichment datasets into their existing customer data profiles via direct integration with mParticle.
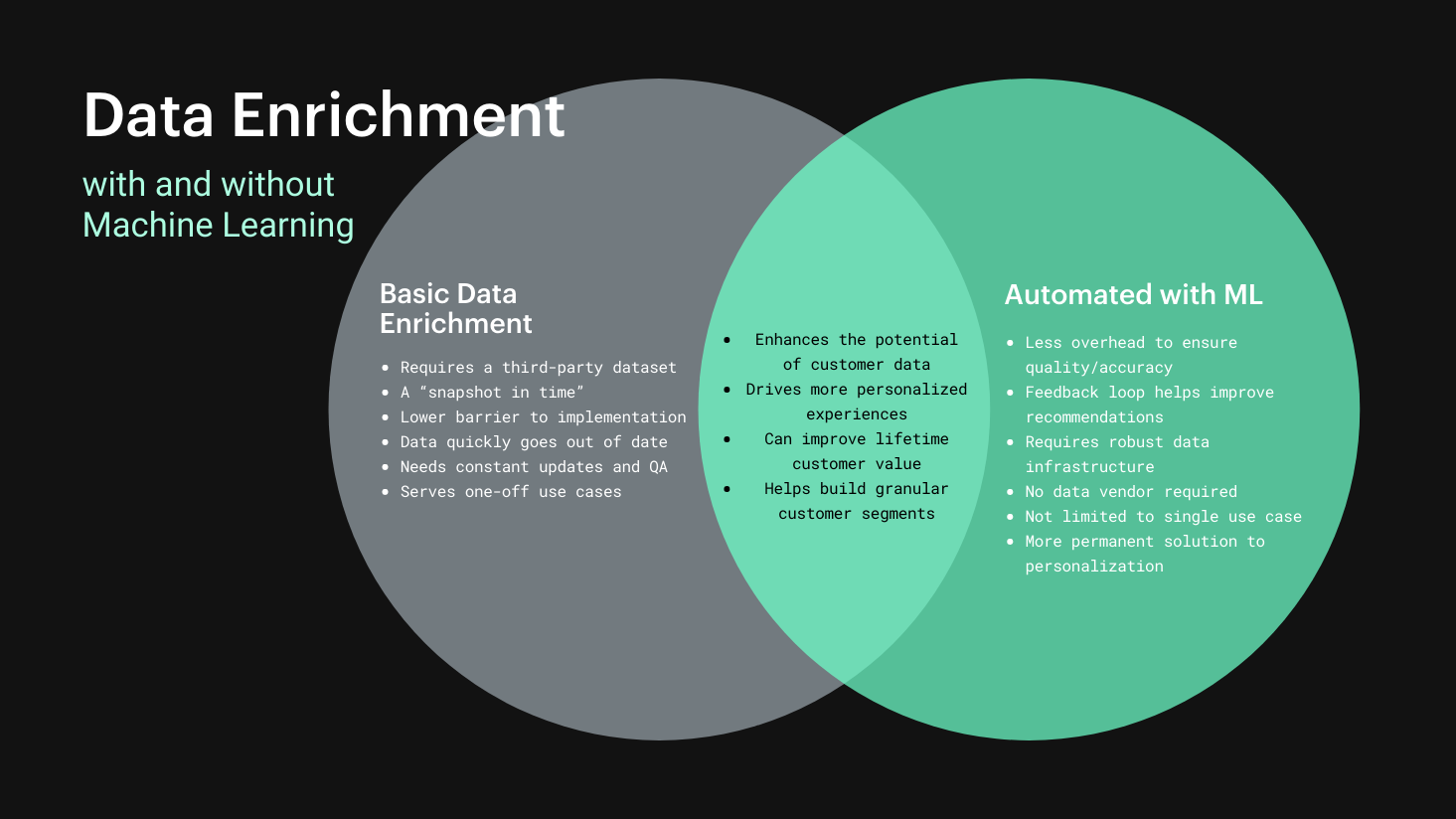
Using machine learning to automate data enrichment
Much like most other aspects of your business’s customer data, data enrichment is not a “set it and forget it” process. Any third-party data set acquired from a partner is a snapshot in time that will quickly become irrelevant as income levels, employers, contact information and other impermanent data points change over time. Outdated information on your customers is worse than useless––it can actually be a significant detriment to your customer relationships if it results in prospects receiving inaccurate and irrelevant messaging. Strategizing on insights gained from your data rather is undoubtedly a better use of time than manually assuring its quality, which is why data stakeholders are now looking for automated ways to keep their customer data as actionable as possible.
Machine learning––a complex and rapidly evolving area within computing––offers one solution to this problem. Thanks to the emergence of tools like AWS Personalize that abstract away many of its most demanding processes, ML is now an accessible way to make customer data more actionable and automate the process of data enrichment without enlisting highly specialized data scientists. Continuously feeding your first-party customer data into a machine learning model will help you draw abstractions and inferences from your customer data without having to manually augment it with third-party datasets.
Overcoming challenges in implementing ML-driven insights
ML is one of the most powerful tools that marketers and product managers currently have at their disposal when it comes to delivering personalized experiences across a company’s touchpoints. While leveraging this pioneering technology for this purpose is attainable, it is not without challenges, the most significant of which being the need to continuously collect and supply quality user data to train and update your model. Without a steady stream of accurate and robust customer data, a machine learning model will not be able to deliver effective insights.
One way to overcome this challenge is to build robust and permanent data infrastructure with a Customer Data Platform (CDP) at its foundation. An infrastructure CDP like mParticle allows you to standardize data collection on all of your digital and offline properties, and organize it into unified customer profiles that are available to every other system in your company’s data and marketing stack. By streamlining the collection and organization of your data, a CDP can both help you supply your ML models with new and accurate data on a continuous basis, and feed these ML-driven insights to the services where they can best be put to use. Finally, a CDP can also help you automate a feedback loop wherein the effectiveness of ML-powered personalization is evaluated and sent back to the ML model on a regular basis, which helps to train the model and improve its recommendations going forward.
Learn more about how you can use mParticle and Amazon Personalize to set up a machine learning model that delivers personalized product recommendations, and automatically improves its accuracy as customers continue to interact with your company’s touchpoints.