Avoiding the growth trap
What do cattle farmers from the 1600s have in common with teams across modern companies? Both rely on shared resources that can quickly be depleted by an overzealous desire for growth, leading to the tragedy of the commons. Learn how you can avoid the growth trap by leveraging your customer data infrastructure and saving your engineering resources from depletion. Stop the vicious cycle, not the development cycle.
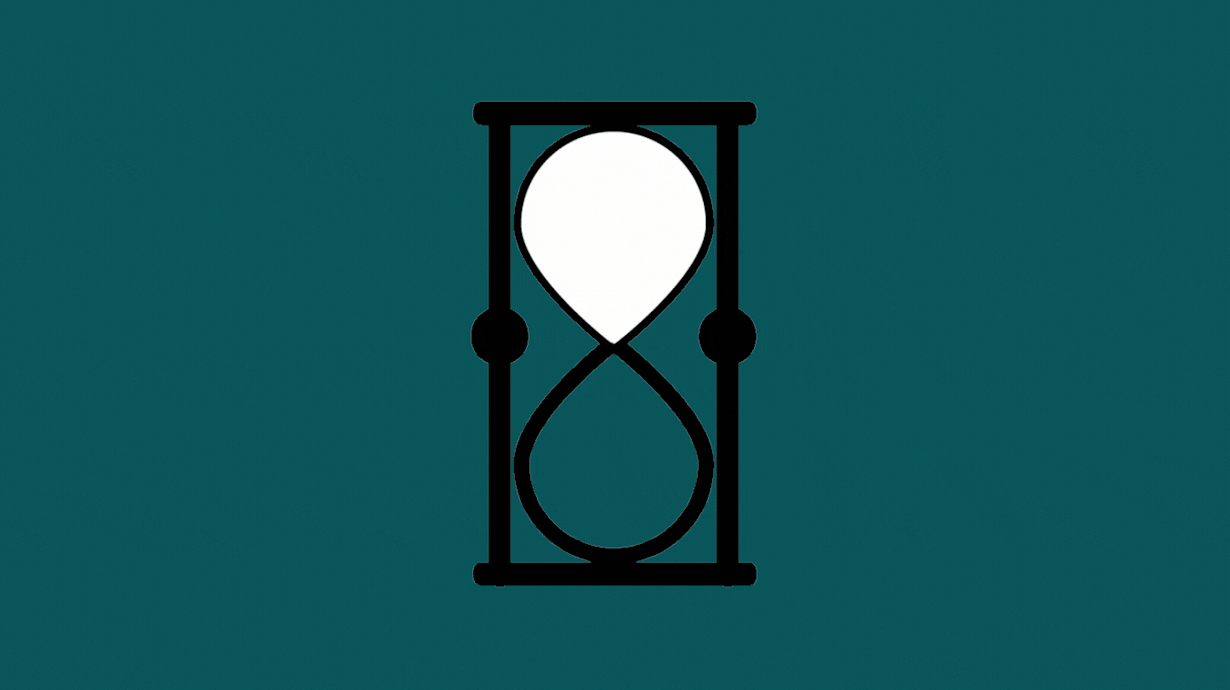
In the 1630s, the fifty-acre plot of land in downtown Boston now known as Boston Commons was a grazing pasture accessible to any cattle farmer. While this was a favorable arrangement initially, the cow grazing ended up causing a collective action problem: Every farmer wanted their cows to consume as much grass as possible so they would fetch a higher market price, forcing the rate of consumption to quickly overtake the pace of new growth. Eventually, the commons ran out of grass, to the detriment of all farmers.
Commons are inherently limited resources shared and used by a group of people. The eventual degradation in quality and ultimate availability which affects all parties due to individual consumption is known as the tragedy of the commons, and any shared resource is vulnerable to this phenomenon. In these situations, the system allows individuals to prioritize resources for their own benefits but doesn’t hold anyone responsible for the collective results of the individual actions, leading to an undesirable collective effect.
While a few things have changed over the past 400 years, teams today often face the same tragedy of the commons dilemma. As COVID-19 thrust every organization into rapid digitization, challenges around personalization, experimentation, privacy, and security became first-order priorities.This priority shift created more demand on a static supply of engineering time with even greater stakes than ever before: ensuring a company's survival.
The engineering commons
Data is a team sport; and on that team, there are data providers and data consumers. Data providers are the Engineers who provide access to data, while data consumers are the various teams who require access to customer data to do their jobs (ex. Marketing, Analytics, Customer Support, etc). Various functions require Engineering time to gain access to customer data to drive growth and will feel the benefit of their demands relatively quickly, but there is a catch: there is no finish line.
Engineering time is a scarce resource that must be carefully considered across the collective demands of the business. Tools and tactics eventually fatigue and the demands continuously evolve, which also leads to non-linear growth in complexity in terms of deployment and maintenance overhead. This compounding effect leads to diminishing returns and accelerates the depletion of important resources required for growth. Much like the cattle owners in Boston during the 1630s, the benefit of the commons eventually erodes, and unfortunately, the end result becomes painful for everyone.
While some may argue that engineering time is renewable, time is not infinitely renewable, opportunity cost cannot be ignored, and regeneration takes time. Burnout is a real phenomenon and, on top of it all, there is no such thing as completion when it comes to engineering. After all, maintenance makes up 60-80% of all software costs.
A shared resource cannot be saved or prevented from depletion without collaboration. The tragedy of the commons arises from the tyranny of small decisions, where a series of small yet rational decisions lead to negative system-wide effects. People, or teams, continue to justify their behavior by rationalizing each decision as too small to make a material impact.
Today, we may see the Marketing team want to onboard a new vendor, which requires access to a subset of customer data in a proprietary spec. The team believes onboarding this new vendor is critical to drive growth so they require engineering time for implementation. But it's not just one vendor for one team, the Analytics team has their customer data needs, the Customer Support team has their customer data needs, along with the executives, business intelligence team, and others. It’s all urgent and it all adds up, and while it leads to initial growth, the resulting growth creates new demands, and the cycle is repeated, until there’s no more engineering time available to the business.
Here is a simple depiction of that scenario. Although in reality there are multiple demands, I simplified it to Marketing and Analytics. Each team makes demands on the Engineering team which does in fact benefit their respective outputs; however, over time there is a counter-balancing impact where diminishing returns are experienced by all groups, representing the natural limits of the resource. The tricky thing is that this counter-balancing phenomenon is not at all apparent until it is, and then everyone is impacted but by then it's too late.
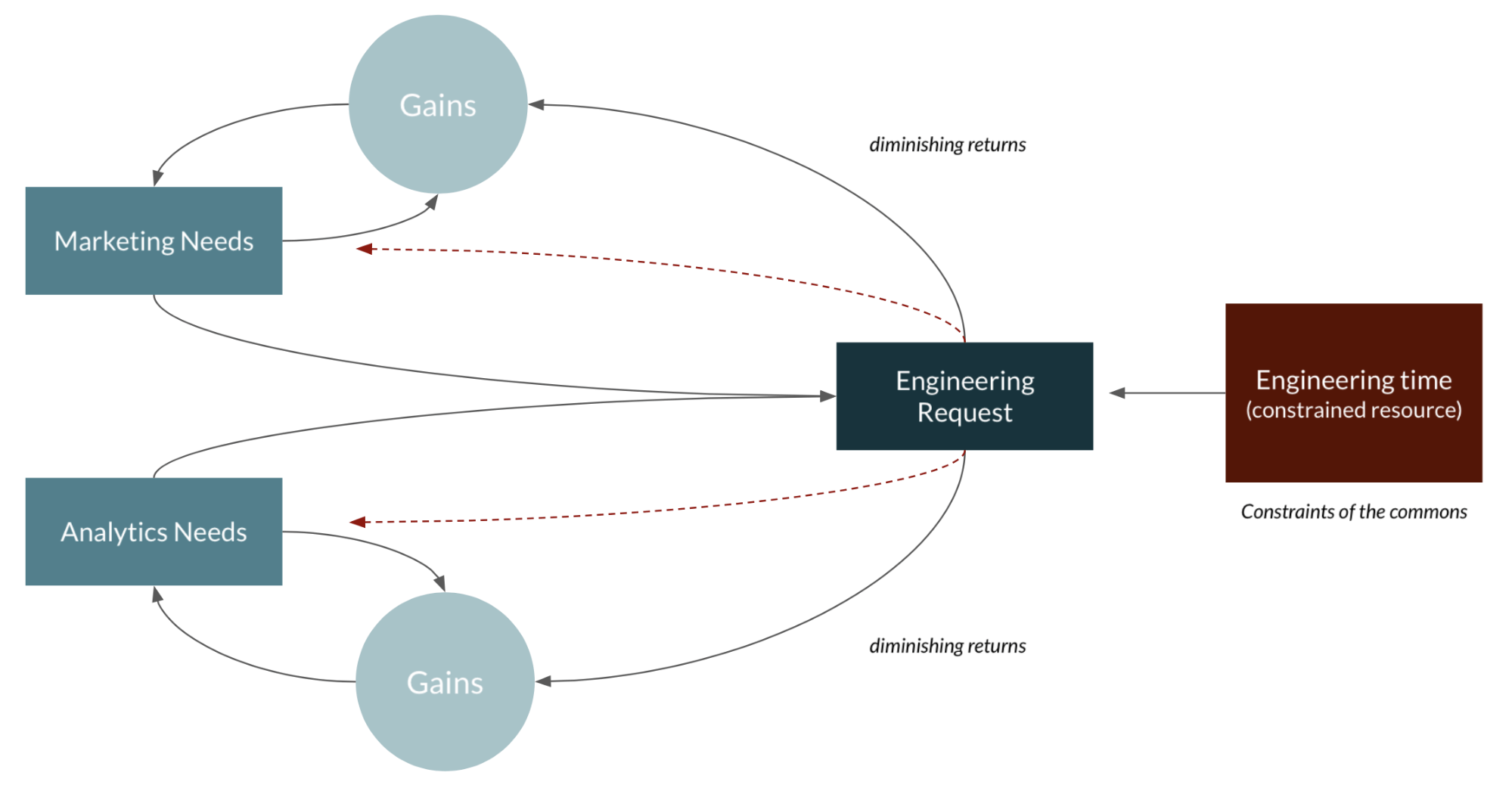
The real problem is that this has initiated a vicious cycle, which means the issues do not end here.
In the example above, a common response is to prioritize one group over another in order to reduce the number of demands placed on the constrained resource. There is a phenomenon called the “digital divide” which refers to those having access to a shared resource, providing them with a significant structural advantage over those who don’t have access. Ultimately, the gap between the groups grows. This is a form of “Success to the successful” which leaves one or many teams at a disadvantage and the organization without equilibrium. The result may lead to underinvestment in critical areas of the business under the guise of risk mitigation and efficiency commonly referred to as “fixes that backfire.” The TL;DR here is that it gets messy fast, and the impact created is non-linear.
Disrupting the vicious cycle, not the development cycle
So how do teams avoid the tragedy of the commons in today's high-growth world?
Teams have three options:
- Avoid the issue (let it get worse)
- Treat the symptom (create other issues)
- Address the problem (the right answer)
Avoidance can take many forms. A few years ago, we would see engineering teams refuse the requests of the business, thankfully that happens far less these days. The form that we most commonly see comes down to a debate whether or not to invest in Customer Data Infrastructure before implementing the next application being demanded by the business or to continue with a tools-first approach. Sometimes this decision comes down to budget constraints, other times the decision is brought into question because of urgent demands being placed on the Product and Engineering team. In either case, this is often accompanied by denial that this approach is detrimental, it's often rationalized as a conservative approach with a ‘wait and see’ justification. The issue is that the problem doesn’t magically go away, and actually gets worse over time.
On treating the symptoms, teams may look at the various implementation demands being placed on Engineering and attempt to use other systems such as Analytics or Marketing Automation tools which have been previously implemented, to integrate data into other systems. This usually ends poorly, where teams attempt to jerry-rig vendors to do things they were never built to do. One issue is that these systems were built to be destinations, not sources; and while these systems offer webhooks to move data to other systems, the real issues are ignored in favor of placing a bandaid on the problem. For example, there may be data coverage gaps, there are most certainly severe limitations around identity resolution, no tooling to protect or improve data quality, and the breadth and depth of integrations is quite limited. By treating the symptom, teams end up ignoring the foundational issues such as data quality, governance, and connectivity required to solve the problem holistically and instead end up spending more time on patchwork that eventually fails anyway.
The final approach focuses on fixing the problem and undoing the vicious cycle. Unfortunately here, there is no easy button. It requires investment in customer data infrastructure.
Using mParticle has allowed us to stay on track with our product roadmap. We no longer need to think about whether or not we have the engineers to implement a new vendor, we can now think more about choosing the right vendor,
Head of Data Engineering at Venmo
To preserve engineering time, multiple stakeholders need to collaborate. Often, the excessive demands placed on engineering stem from organizations having a hyper-reactive approach to data consumption in an ever-changing privacy, regulatory, and SaaS environment.
The solution begins with the formulation of a customer data strategy, a comprehensive identity framework, and a lean tagging approach that is predicated on the intent to distribute customer data across a multitude of vendors in real-time. Only through the investment in Customer Data Infrastructure can teams protect their engineers and avoid the growth trap.