Improve products with AI and Machine Learning
Traditional digital marketing can’t keep up with machine learning and AI marketing solutions. Learn exactly how these powerful point-and-click tools work and how they can help your team experiment at scale.
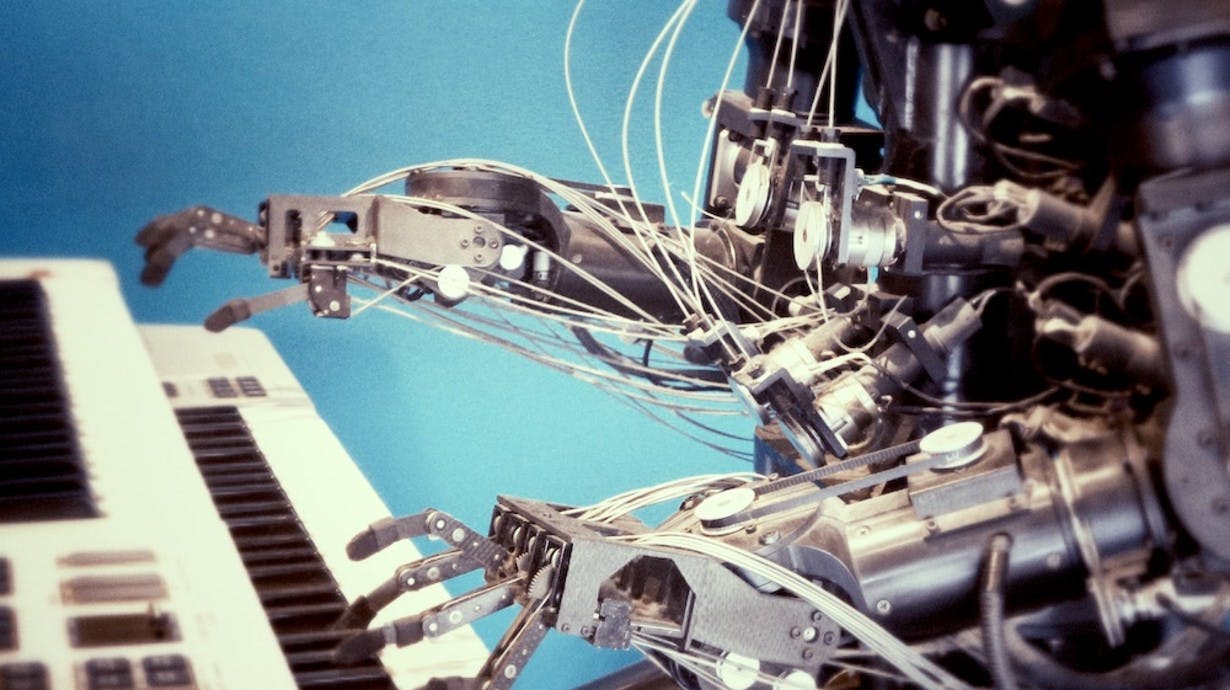
Introduction
AI and the data-backed decision advantage
The ideal customer experience (CX) occurs when customer expectations perfectly match the real world experience. Customer expectations have continued to rise as companies personalize and streamline experiences at every touch point, making it critical for modern brands to optimize their product and customer journey should they want to acquire and retain customers accustomed to the near-perfect CX scoring experiences created by Netflix, Airbnb, Uber, Amazon, and Spotify, among others. Modern organizations rely on their product and marketing teams to create the products, features, and workflows that deliver the best customer experiences. In an ideal world, product teams are free to create and execute a product roadmap that introduces differentiating features and improved end-user experiences based on customer’s needs and feedback. Unfortunately, product teams often spend a significant portion of their time working to address bugs and other issues, implementing and maintaining SDKs, and responding to ad-hoc requests for data. Without additional headcount, organizations can end up with a product team that is constantly playing catchup rather than delivering new and optimized features that will make them stand out from their competitors.
So, how can brands deliver an optimized UX, improve agility, maintain their roadmap, and accurately map KPIs without expanding headcount? Product and marketing teams can both benefit from leveraging machine learning and artificial intelligence to test and deploy personalized experiences based on real-time and historical engagement data.
In this blog, you’ll learn how machine learning and artificial intelligence (AI) breaks down into Four Stages of Predictive Personalization, and how companies like Overstock have used AI to exponentially improve product output and marketing efforts, as well as some practical tips on how to get started with available AI methods in your organization.
The UX divide has narrowed
Customer experiences should be personal, predictive, occur in real time, and seamless across the ever-increasing number of devices and platforms used by modern customers. In the past, digital marketing focused mostly on acquiring new users and driving them to purchase. Now the entire customer journey is in product and marketers’ hands, from acquiring new leads to cultivating lasting relationships.
The platforms, devices, and channels through which customers engage with a brand’s digital properties generate a near-infinite number of conversion variables which introduced additional complexities that left product teams with mass amounts of data to parse through to gain insight into the end-user experience.
Before AI, most brands could not keep up due to:
- Device quantity explosion
- Voice considerations
- Regulatory restrictions / GDPR
- Cloud proliferation
- Scarcity of developers
- Massive data scale
Current machine learning tools enable brands to deliver an experience that rivals industry leaders, while minimizing the amount of time and manual labor needed for integration, maintenance, and analysis. AI democratizes digital marketing, which means many companies can now:
- Find the next customer from current customers.
- Engage customers on any screen
- Gain insight into every interaction across a customer’s journey
- Have machines do the grunt work—at scale.
- Be increasingly agile and iterative
- Free engineers and product teams to focus on core business problems.
According to Gartner, as marketing matures, leaders will invest in multichannel, multidisciplinary technologies that promise enhanced, real-time decision making based on real-time data, machine learning and artificial intelligence (AI). AI will be used to deliver data-driven marketing insights and automate channel selection, campaign orchestration, optimization and measurement.
The four stages of predictive personalization
The objective of predictive personalization is to give the right message or content, at the right time, in the right place. This occurs in four stages: Instrumentation, Segmentation, Customized Recommendations, and Personalization.
Stage 1: Instrumentation
This foundational layer affects everything else downstream. It all starts with identifying actual business KPIs that you want to optimize. The five key buckets are:
- eCommerce - products, promotions, impressions, etc.
- Lifecycle - telemetry, sessions, starts/stops.
- User - user IDs, user attributes, household IDs.
- Custom Events - behavioral interactions, user interactions (search, navigation, purchase, etc.) - what drives conversion.
- Screens & Pages - screen info, pages, pathing.
KPI data is collected and unified from all sources (SDK, cloud feeds, API, CSV) by a customer data layer, like a CDP, then mapped to a standard data model. This establishes a clear customer ID and behavior patterns across devices.
Centralized and standardized data can then be pushed out to where you need it. For example:
- Event integrations can stream raw data to analytics to track and analyze any user interaction.
- Define dynamic user segments and orchestrate experiences across platforms and channels.
- Data can be synced with EDW or Data Lake in real time.
From first touch to conversion, tools like mParticle can instrument the data to the correct format that a machine learning model can then understand. This initial data prep is elemental to unlock the full potential of AI.
Stage 2: Segmentation
Basic, non-AI segmentation involves a very limited selection of attributes and static inputs. Predictive segmentation, however, lets you analyze thousands of attributes and implement a dynamic user scoring.
Let’s take the add to cart message. In the past, you would retarget customers based on intuition and limited parameters. With predictive models, your analysis incorporates 1000s of attributes. You can even weigh multiple permutations that vary according to location, time and other factors.
To capture all the possible permutations, tools like Clearbrain implement AI to automatically adjust the data into machine-readable format. The result is an automated platform that makes data manageable and easy to understand.
On the user side, you have a point-and-click interface to identify conversion events. The model automatically looks into past data to see who added to cart and who didn’t. This information is then compared to the individual’s attributes and behaviors and each is weighted appropriately.
The result is a prioritized list of users based on their probability to do something you want them to do. Instead of limited data, guesswork, and hunches, you have weighted data to guide marketing investment intelligently.
Stage 3: Customized recommendations
Once you have a predictive segment list of users, each user can then be scored. Still, even though you have the data, you need to coax them along the buyer journey.
In the predictive personalization pipeline, there are three actions you can choose from once you finish user segmentation:
- Recommend an inclusion or exclusion group - For a Facebook audience ad group, you could exclude high probability converters since your ad dollars will be wasted on them. Also, on the low probability end, they click but never convert. The middle ground is where you should focus since you can influence their behavior and max out ad ROI.
- Recommend different content - Since you can predict which users are most likely to add to cart, you can now recommend emails, ads, or even on-site personalization that matches their preference. Instead of showing an entire catalog, it’s personalized. This even takes into account if they’re more likely to buy in the afternoon vs. the evening.
- Recommend different pricing - Discounts are similar to ads. For high probability converters, you avoid discounts as they cut revenue. Develop proportional discounts to optimize price per conversion.
Stage 4: Personalization
While in Stage 3 you made the choices, Stage 4 allows you to sink these decisions into platforms. For example, you can:
- Personalize ad audiences on FB or Google Ads.
- Sync scores into a tool like Optimizely for A/B testing.
- Personalize the on-site experience by recommending different content, discounts, coupons or email platforms.
The result is an end-to-end automated experience at the user level rather than the audience level. You predict what they actually want or need and at what time. Thanks to machine learning, this process occurs in minutes, not months. Plus, it would take years to build a similar solution in-house.
The richness of the data enables you to prove which audiences drive lift by channel. This allows you to maximize the ROI on ads, emails, and push campaigns.
Less GDPR risk
Since we’re now in a post-GDPR ecosystem, ad strategies based on third-party data enrichment may put your brand at risk. Machine learning allows you to leverage first-party data in a new way as you extract audience segments, attributes and user properties from your own proprietary data. With less reliance on data brokers, you minimize regulatory risk.
How Overstock uses machine learning to personalize customer experiences
Overstock has remained a relevant retailer for over two decades demonstrating consistent durability and growth while striving to constantly improve the user experience (UX). As a publicly traded entity and member of the Russell 2000, the company’s annual revenue currently tops $1.8B. But, like many large enterprises, Overstock managed many marketing channels that were non-integrated and segmented by audience rather than individuals. The lack of marketing personalization resulted in lower efficiency, suboptimal UX, and millions of dollars in excess ad spend.
Overstock sought a solution that would unify their marketing vision across all channels to provide the most personalized user experience possible without placing an undue burden on their product and engineering departments. They required a versatile strategy for all elements to be able to speak to each other, create exceptional UX, minimize cost, and drive revenue growth. After much consideration, the team at Overstock decided to invest in a three-part stack, consisting of a machine learning provider (Databricks), a data warehouse (Snowflake), and a customer data layer (mParticle).
Before selecting mParticle as their foundational customer data layer, Overstock made a list of requirements to ensure they would create a marketing tech stack that best suited their current and expected future needs. Their criteria included:
- Rapid Deployment - Overstock needed to be able to quickly develop and deploy new models that would define product and marketing initiatives.
- Future-proof - Modular, accessible, agnostic, best-in-class stack component
- Real Time Ready - Able to collect and activate in real time, knowing that there is no time or budget available to rebuild a stack gone wrong.
- Cross-channel - Able to collect, connect, and activate data across many platforms and devices
- Fast Integration - Be able to quickly integrate new tools and improve time to value for marketing and product initiatives without relying on engineering
- Machine Learning Able - Increase the speed of deployment and accuracy of machine learning models, unlike on-premise methods which took weeks to ramp up.
With mParticle, Overstock implemented real-time personalization and coordination across their many marketing pieces, such as email, API based campaigns, infrastructure, content, UX, promotions and advertising.
The resultant single, organized view delivered upon the personalized one-to-one experience promise. Machine learning gathers it all in from a wide range of contexts, devices and channels to unify and accelerate processes.
Overstock's new and improved machine
Overstock’s new marketing tech stack needed to be capable of continuously collecting clean, individual customer data from every touch point to feed machine learning models and act on the results in real time. Choosing mParticle as their customer data layer not only gave them a way to collect and access their customers’ engagement data, it also provided them with a way to quickly and easily integrate new tools through mParticle’s API to ensure that this data could be analyzed and connected across their stack for modeling and subsequent activation, without the need for additional third-party code.
This is how it works: Raw user-level data comes in through mParticle, is forwarded to Snowflake where it is rolled up into features, the features are sent to Databricks as model inputs and model outputs come back to mParticle through AWS Firehose as user attributes, which are then forwarded to ad platforms, email providers, social platforms, and beyond. When customers take actions on the site or open an email, for example, those data points are collected and ingested to build a continuously-updating user profile that enables Overstock to tailor messaging and displays towards individual customers’ preferences and informs ad bidding in real time.
Putting this stack into place provided Overstock’s marketing and product teams with many benefits, including:
- Unification - Identifies customers across many contexts, devices and events.
- Plug-n-play - Rapid deployment transformed company marketing stack in 8 months.
- Ad-vantage - Increased leverage of acquisition and arbitrage in ad opportunities.
- Single Data Stream - Eliminated most data engineering needs.
- Hyper Testing - Exponentially increased testing capacity.
Key models developed
By implementing mParticle, Overstock subsequently developed several growth driving models, such as:
- Time of Day - Identifies the ideal time to contact each consumer via push message, email or RDSP.
- Channel Propensity - Determines where they’re most likely to interact. Enables audience refinement and accuracy.
- Expected Purchase Value - Helps determine how much to bid on paid channels. Can group people into custom audiences of similar ad impression value.
- Category and Product Affinity - Custom email content by category, products, etc. personalized to each user.
Customer acquisition dollar efficiency increases dramatically when you have arbitrage opportunities, and these are only available for short periods of time. Therefore, systems must be sophisticated and fast enough to gain an edge. Models like Expected Purchase Value demonstrate this advantage.
Results
In the time Overstock has been using this stack they have seen:
- An overall 10% increase in advertising efficiency across the marketing department in the first eight months.
- The data model deployment cycle has decreased from an average of three months per model to one day.
- Reduction in launch cycle for vendors from being measured in months to being measured in days. For example, Overstock was able to integrate Braze into their stack in 8 days vs. 1-2 years using conventional methods.
- Highly-tailored email messaging and improved recommendations for customers based on their preferences Improvement in conversion rate and resource consumption
- Lowered API dependencies and higher system uptime
- Highly accessible and structured data
- Increased ability to test across content, channels, audiences, and platforms and increased percentage of known users identified on multiple channels.
Machine learning now
Overstock now commands a single stream of data produced from all channels. This eliminates most data engineering needs when pushed into machine learning features.
For instance, as most tests are inadequate, automated testing gets the right answers faster. Consistent data structures make this possible, and faster testing means faster growth. Instead of a few tests built on hunches, thousands can be run simultaneously.
Also, data from mParticle gets streamed to the Overstock data warehouse where automated feature roll ups are located. A pool of features are then built, and hundreds of features become available to any model. Later, from the data warehouse, machine learning pulls features and writes back outputs to mParticle as an attribute.
Now the company knows - with much greater precision - when to send an email, what content to show, when to recommend a loyalty program, what channel to advertise on, etc., as it’s all highly personalized. This optimizes channel use and can even be price adjusted for users and ad bidding strategies.
Wider vision
The biggest impact machine learning has had on the company, perhaps, is a changed mindset. Organizational silos have broken down, and teams think more holistically. A database of all users that ever visited their site, all tagged with associated attributes and events, opens up tremendous possibilities that the company continues to explore.
At Overstock, the marketing strategy for the next three years is clearly visible. The company will continue to invest in identity refinement and strive to deliver the best customer experience on Earth.
Practical Q&A: Getting started with AI tools
What are the conversion events I should choose to optimize first?
Map out your customer journey from first touch to conversion, then choose which event is most interesting or valuable to you. Begin there. The larger the sample size and the more digital signals the better. Sample sizes starting at around 25-50k users work best.
Predictive models are perfect for mid-market, medium-large sized, high digital engagement companies. In some cases, B2B may not work as well since the lag time between touch points is longer. Also, B2B depends a lot on the first touchpoint which may not have a strong digital signal.
What’s more important: getting new acquisitions or focusing on existing users?
It makes sense to activate on existing users first. Look at what’s happening now and optimize it. You can check activation, upsell, re-engagement, etc., and build predictive audiences around those areas.
Find out who’s most likely to become inactive, turn, cross-sell or upsell. Build predictive audiences, and automate workflows around them, such as an email drip campaign, email retargeting or post-funnel experience automation.
What if I’m still not sure about what to automate?
When in doubt, just point-and-click and within minutes you have data about different conversion events. Machine learning weighs every previous behavior and attribute to assess relative importance. One example is user churn. Instead of segmenting for churn, look at the upstream events before churn appears. This way you can optimize to minimize churn.
Start your AI journey
Creating customer experiences that not only meet, but exceed, customer expectations requires a significant understanding of what customers want and how to deliver it to them when and where it matters most. If you'd like to learn more about how mParticle can help you leverage machine learning and AI to create experiments at scale and improve your product output, get in touch with one of our experts here!